7 Steps to Data-Driven Success: Transform Data Management and Accelerate Digital Business Operations
Data – the element that fuels digital business – is often taken for granted. Bad data, like bad fuel, can clog or block distribution and leave a business stranded. Make data good and fuel your digital business.
Becoming a Data-driven Organization is Difficult
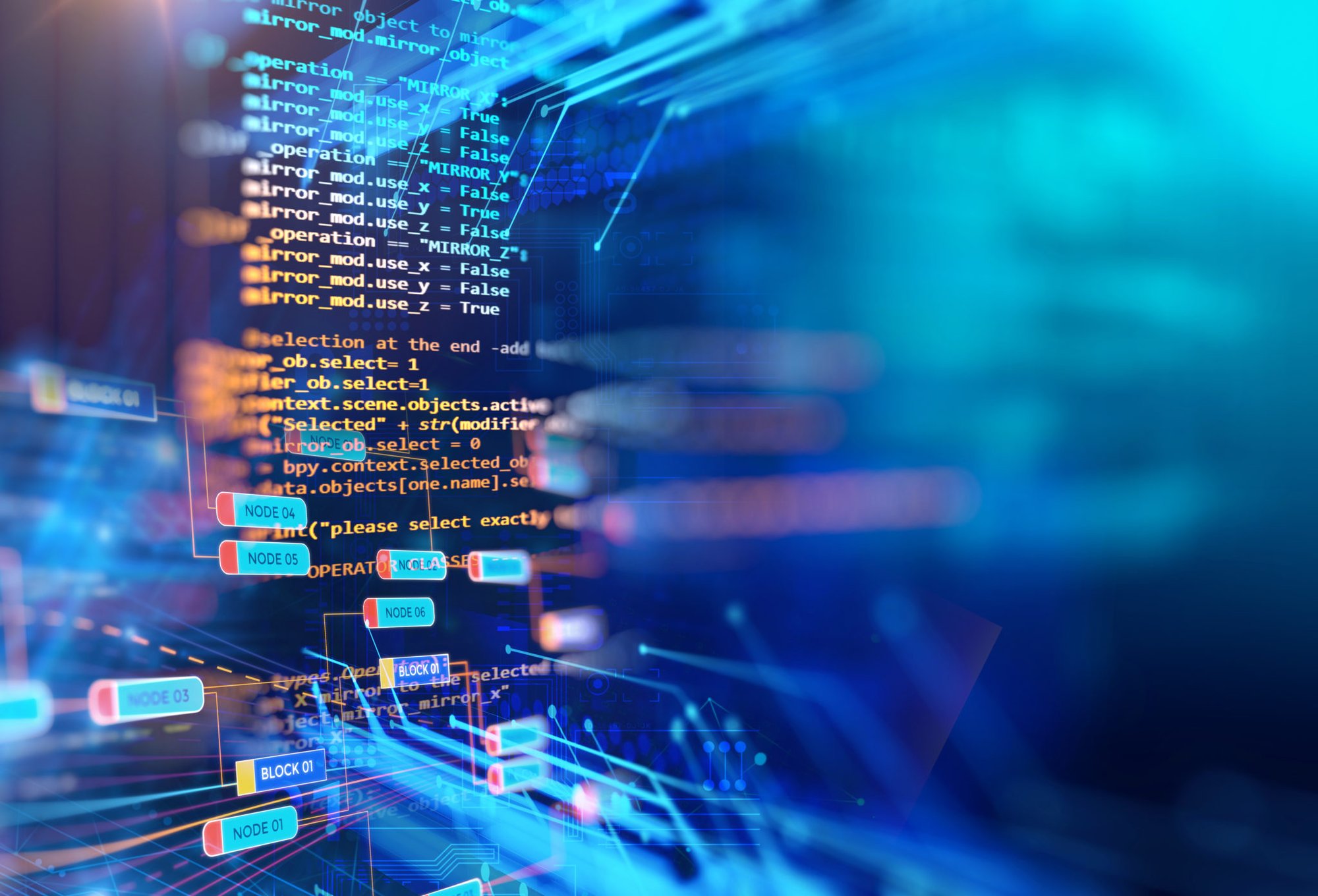
Changing how people do their work is why “transformation is a long-term process that requires patience, fortitude, and focus”, says Bean..
Transforming an organization to be data-driven requires changes to the way people use data in their work, and operational processes, by introducing digital processes and practices. Nowhere is this more important than how a company handles its data, including in its applications. At its core, it requires changing application-centric business operations to data-driven ones.
Data-driven operations are fueled by data. Every company believes its data is “good” because data is utilized to run the business. How can data be bad if a company’s accounts balance and its transactions are processed successfully? It can be bad when it is data not fit for its purpose. For example, data may not be complete enough to satisfy a customer’s need in real time, or available for a management inquiry or for a financial analysis. Data requires work to make it usable.
The Problem of ‘Bad’ Data
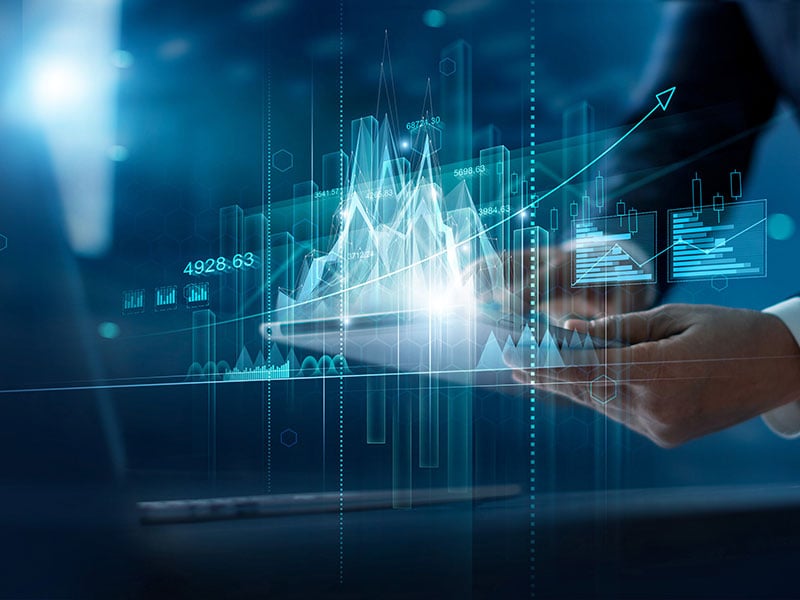
It’s data that you can’t access, data that you had and then lost, data that’s not the same today as it was yesterday… In short, Bad Data is data that gets in the way.”2
What digital business process or analytic can afford to use bad data? Digital businesses can’t operate using data that gets in the way – automated processes and activities require good data to increase business efficiency, efficacy, and agility.
Good data is more than data quality. Data quality targets accuracy, completeness, consistency, reliability, and currentness of data as its core concerns. While data quality is important and necessary, focusing only on these characteristics of data does not consider how data is being used. Applications or individual work by data scientists and business analysts use data for their own purposes.
Presenting ready-to-use data in real-time to customers, workers, partners, and others, separately from their applications, is necessary for digital business. Data where you need it and ready to use when you need it requires good data throughout the business. Data usable in real-time, independently of applications, is essential for digital success.
Seven Steps to Data-Driven Success
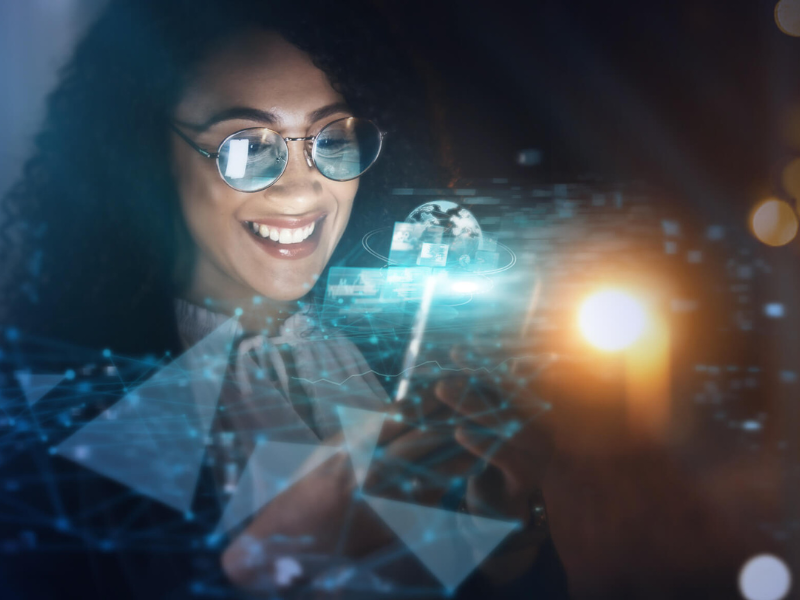
The focus changes from operational work to data use and automation. The steps to data-driven success presented here provide a guide for enabling data-driven operations.
The seven steps are in three categories:
- Understanding Data Use, with a strategy for data use and personalized data interactions
- Data Use Data Management, with data localization, data organization for user needs, and data delivery automation
- Data-Driven Operations, by building applications focused on data delivery and real-time data and automation
Step 1: Form a Strategy for Real-Time Data Use
Many companies have data strategies. A data strategy is “a coherent strategy for organizing, governing, analyzing, and deploying an organization’s information assets.”3 This framing is important for protecting and leveraging data assets in general, where a data use strategy is a strategy for managing enterprise data – data that exists in applications but is used outside of them – and effectively acquiring, storing, and delivering it to serve enterprise purposes.
Digital and data-driven operations focus on data use. It changes operations from how people do tasks to how data and automation enable quick, often in real-time, interactions, decisions, and services. It begins with establishing a strategy for enabling the utility of data throughout the enterprise. A data use strategy identifies data content used independently of enterprise record-keeping, makes it good in the most effective way, and maximizes its usability across the business. Having digital data ready to use in real-time anywhere in a business requires data that is captured accurately, completely, consistently, and reliably at its initial point of capture, and used consistently everywhere from that point forward throughout the business.
Data content is typically the responsibility of each application capturing it. Making data use a strategic consideration requires enterprise data considerations to be an important element when building, buying, or renting an application. However, applications are product-centric, in that data is centered on the product or service the application supports rather than centered on a customer, partner, or worker. Or, like Salesforce or Workday, applications are centered on a worker rather than a customer, partner, or product.
A data use strategy recognizes that the enterprise has data needs as well as functional application needs, and both need to be addressed at the time an application is being selected. Instead of data being treated as ‘exhaust’ from applications, data is treated as enterprise fuel for digital business. Application data capture acts as a good or not-so-good fuel filter. Methods to make data good and ready to use can be implemented along with the application, which will also benefit from ensuring the data it captures is good.
Digital changes the focal point of data planning from internal record-keeping to data users. A way to think of this is from outside the business in instead of from inside the business first.
Data Use Strategy Action Items
Purpose
Identify enterprise data content that needs to be ready-to-use in real-time for specific data-driven objectives (don’t boil the ocean, but do set the stage for the following actions)
Action Items
- Establish an operational focus for data use by identifying the scope of the Data Use Strategy:
- Categories of users who will consume data-driven information, and their roles
- Data scope, which organizations may call ‘data subject areas’ or ‘data domains’, such as worker, customer, organization, and so forth, to provide a high-level view of the data needs of each user category and role
- Establish data-driven objectives by identifying the key metrics and operational actions they are to drive
- Create a business term glossary containing data definitions and validation rules for consistency of understanding and use, and developing “data literacy”
- Identify areas of automation needed for data-driven operations:
- Data preparation and redundancy elimination (this will be detailed in Step 4: Organize Data)
- Machine learning and AI opportunities for data-driven automation
Step 2: Personalize Every Data Interaction
The world today is shaped by experience with mobile devices. We are used to seeing what we want when we want to see it, and we expect the content to be relevant to our interests. Use of mobile and web platforms is also shaping business applications. It is the intersection of an individual user and the digital business data where the two sides of personalization come in.
Personalization is generally understood as taking information about an individual and tailoring a service, product, experience, or communication based on it. Data personalization is less about individual tailoring and more about understanding users of, and their use of, data so it can be ready for their use. Data is personalized when it can support the perspective of each user role and provide content that satisfies that perspective in real-time. Data use that maximizes users’ experience cannot succeed with a “one size fits all” approach.
For example, a customer perspective organizes all customer-related information by customer, making it customer-centric data. Similarly, a partner perspective organizes information as partner-centric, and a worker perspective organizes information as worker-centric. The essence of each perspective is that “it’s all about me” – i.e., it’s personalized to the user’s role.
Digital business presents data content to users from their perspectives in real time. Personalized data content is what the user interface needs to contain for a user. Rules-based decisions, machine learning, and AI also use data differently than applications, so data content for automation needs “personalization” to support real-time automation. Digital data use is different than applications’ data use, particularly when users are allowed to change data and initiate transactions with a mobile app. This creates challenges for the organization and structure of data.
Data Personalization Action Items
Purpose
Identify the data content needed to execute data-driven actions for each user category and role identified in the Data Use Strategy
Action Items
- Personalize data by identifying for each user category and role:
- The data and analytics content needed
- Mechanisms, such as a website, mobile app, or application, the user role uses to interact with data content
- Key metrics and operational actions they are to drive
- Automation needed for data-driven operations
- Add new data elements to the business term glossary containing data definitions and validation rules for consistency of understanding and use
Step 3: Localize Data for Its Use
As digital business drives to mobile interactions with customers, partners, and workers, location of data becomes important. At one level, it is making data available to interact with users in real-time, but where data resides is also a digital business issue. Global businesses need to comply with regulations some countries have which govern where data about their citizens and residents must be stored. This means knowing where data originated, who provided it, even when it was provided, and therefore what and where it must be stored.
The physical distribution of data is a critical issue for digital data management. Data today is in the cloud and intended to be available to respond to users’ needs in real-time. If users are wide-spread, data location may need to be regionalized to reduce latency in data delivery.
For a digital business to be successful, data localization, like data personalization, is a factor that must be incorporated into real-time physical data management. It encompasses data privacy regulations like GDPR, CCPA, and others, as well as where data can be physically stored. It accentuates the need for digital businesses to look at their operations from the outside world in, instead of from their applications and data stores out to the world.
Data Localization Action Items
Purpose
Identify the regulatory and operational requirements for user categories’ data content, such as adhering to HIPAA, PCI, PII, GDPR, CCPA, country limitations on storing data, and corporate policies
Action Items
Identify for all data content:
- Regulations and corporate policies that are to be applied
- Review with corporate Compliance and InfoSec for approval
- Document compliance enforcement plans, including automation
Step 4: Organize Data for User Needs
Digital business needs ready-to-use data for automation and real-time interactions. Data needs to be organized and structured, so it can serve all these needs.
Enterprise data planning focuses on core business entities, such as customers, partners, workers, products, and so forth. Data planning begins with identifying, for each business entity, where its data enters the business and controlling it at one point of data entry. Instead of each application, for example, capturing its own version of customer data, create one customer set up and validation service that each application will use by interacting via APIs. Make customer set up and validation a service for maintaining customer core data in a current and correct form and its system of record (SOR).
End-to-end data management focuses on sustaining consistent and correct data throughout a business and its use. The “end” to start with in end-to-end data management is the user’s because maximizing each user’s experience is the mission of digital business. This requires data to be organized to support the needs of data users with whom the business interacts digitally, not structured by applications the business uses.
Data organization is not the same as data architecture. “Data architecture describes the structure of an organization’s logical and physical data assets and data management resources, according to The Open Group Architecture Framework (TOGAF).”5 A focus on data as “logical and physical data assets” is well-suited to static data, where an application’s physical data does not change often, and a logical data model represents the abstract language of a business.
Data-driven decisions and automation are more dynamic. User needs and uses of data are not static but can change frequently. Organization of data for digital business is both granular and aggregated and is dictated by the requirement to deliver them in real-time. Data architecture organizes data as entities and tables typically governed by relational normalization rules. This is like chemical elements in the periodic table that are organized by an element’s atomic weight and electron configuration. It is useful as a structure of information, but not for representing integrated content, such as for material science or engineering uses.
Similarly, organizing data for user needs requires integrated content specific to a use. In today’s technical architectures, data interactions are becoming precise and executed using APIs. This requires a usage-driven data design approach for configuring data instead of a relational data architecture.
Direct and streaming data exchange are changing data architecture from organizing data into relational tables as an enterprise data architecture. APIs are a mechanism for data interactions using JSON notation, which make JSON objects better suited to data organization today.
The fundamental trend is for real-time interactions using consistent and correct data. This has implications for future applications as digital businesses become event-driven and work to eliminate latency between an interaction and providing a response.
Data Organization Action Items
Purpose
Organize and structure data content so all user categories and roles, and personalization and localization specifications can be satisfied in real time
Action Items
- Organize data by specifying for each user role:
- The data content of each UI element for the data and analytics a user will interact with
- Mechanisms, such as a website, mobile app, or application, a user role will use to access data content, which may duplicate or add user UI elements
- Key metrics and operational actions UI interactions are to drive
- Structure data by using the data content of the UI interactions (create a usage-driven data design, not a data architecture) and:
- Performing an affinity analysis of the data content to determine the distinct data objects / data element groupings the UIs require
- Analyzing data access paths to determine the relationship linkages between data objects
Step 5: Automate Data Delivery and Analytics
Real-time, event-driven, personalized, and localized interactions are fast becoming the standard for data processing in a digital business. Managing these aspects requires Data Use Data Management. The standard definition of data management is that it “consists of the practices, architectural techniques, and tools for achieving consistent access to and delivery of data across the spectrum of data subject areas and data structure types in the enterprise, to meet the data consumption requirements of all applications and business processes.”4
Data use and automation have different data management concerns, from regulations and compliance to real-time consistency across user interaction methods. This is the heart of digital data engineering – fueling a business so it can respond to users in real time without data issues getting in the way. Data automation means updating data programmatically rather than manually.
Keeping data correct and consistent throughout a business requires automation focused on systemic enterprise data instead of individual data flows and pipelines between applications and analytics. Individual pipelines are susceptible to unannounced or unexpected changes in a source application.
This has implications for business applications and sources of record (SOR). Transactions no longer originate only in an SOR when a user can initiate them using a mobile app. The SOR remains the processor of (and SOR for) the transaction but, from the perspective of mobile app users, their transactions are underway, and they can move on to other things.
Automating data means systemic, end-to-end data management, from origination-to-archiving, instead of an application-centered view of data. Digital businesses control the capture of data, manage its use, and ensure that SORs can do their job and that data can be personalized, localized, and automated for real time presentation to users. This makes enterprise data planning an essential activity.
Because users will continually develop new data needs and interactions to satisfy, it also makes keeping data organization, physical structures, and APIs up to date a sizable challenge. IT automation is necessary if a company is to really achieve real time and data driven business operations.
Data Delivery Automation Action Items
Purpose
Identify the automation opportunities and patterns for automating data and analytics content
Action Items
Plan for implementing data automation by:
- Identifying the SOR for each data element of the data content and all SOR data consolidation and synchronization requirements
- Defining the strategy for keeping data use, analytics, and SORs consistent when user interactions can add, change, or delete data (a basic feature of mobile and web applications)
- Specifying website, mobile app, or application channels of data use so consistent and complete data is delivered across all of them
- Specifying data services for ingesting data into, and publishing data from, the stored data and analytics content
- Establishing a process to manage stored data and analytics content
- Automate the data structuring process, incorporating governance and data rules, and manage the evolution of data, analytics, and UIs and their underlying data design
Step 6: Build ‘Outside-In’ Applications
Businesses that are data-driven change from being application-centric – acquiring operational functionality as the primary criteria – to being data-centric and prioritizing data and its delivery to users with little or no additional processing. Data is raw material that needs to be refined for business use in real-time. A top objective for real-time data is eliminating latency in capturing, preparing, and using data for digital purposes. ‘Outside-in’ development means designing an application based on its user interface and data interactions. Like end-to-end data management, start with end-user roles as the first step in design of user interactions and their underlying data structure. This outside-in approach can also apply to application development.
Users are best served when data definitions, formats, content, correctness, consistency, and currentness are the same wherever and whenever data is used and interacted with. The legacy view of a business application is as a self-contained set of programs and databases designed and built that, collectively, don’t serve users with consistent and correct data.
An approach to the problem of applications and shared data is microservice architecture. “A microservice architecture – a variant of the service-oriented architecture structural style – arranges an application as a collection of loosely-coupled services. In a microservices architecture, services are fine-grained, and the protocols are lightweight.”6 A true microservice has each service responsible for its own data, and no other service outside the specific domain directly interacts with that data.
A challenge with microservice architecture is maintaining data consistency. “In order to ensure loose coupling, each service has its own database. Maintaining data consistency between services is a challenge because 2 phase-commit/distributed transactions is not an option for many applications.”7 A microservice is meant to fulfill a single responsibility, so reading, writing, and updating data are individual services for each responsibility acting on a single data domain.
Digital business needs data to be ready-to-use for operational automation, so it comes down to data and its intersection with data stored in disparate applications and data domains. From the perspective of data use, supporting a data interaction requires microservice discovery and orchestration. Digital business cannot afford the latency required to make such data consistent and correct for use in real time.
I believe it is better to view an application as UIs and data interactions personalized, localized, and organized for users. This enables end-to-end data management by focusing application development on user, including application users’ interactions with data, and separately focusing on data content supporting applications and data delivery and analytics.
Treat applications as real-time user data use that are also ready-to-use in real time for data-driven objectives.
‘Outside-In’ Applications Action Items
Purpose
Develop applications that eliminate duplication of data and rules and the associated data inconsistencies they cause
Action Items
- Implement outside-in applications by identifying:
- Categories of users who will consume data-driven information, and their roles
- Data scope, which organizations may call ‘data subject areas’ or ‘data domains’, such as worker, customer, organization, and so forth, to provide a high-level view of the data needs of each user category and role
- The data and analytics content needed
- Mechanisms, such as a website, mobile app, or application, the user role uses to interact with data content
- Key metrics and operational actions they are to drive
- Automation needed for data-driven operations
- Identify for all data content:
- Regulations and corporate policies that are to be applied
- Review with corporate Compliance and InfoSec for approval
- Document compliance enforcement plans, including automation
- Organize data by specifying for each user role:
- The data content of each UI element for the data and analytics a user will interact with
- Mechanisms, such as a website, mobile app, or application, a user role will use to access data content, which may duplicate or add user UI elements
- Key metrics and operational actions UI interactions are to drive
- Structure data by using the data content of the UI interactions (create a usage-driven data design, not a data architecture)
Step 7: Commit to Real-Time Data Operations and Automation
Even if a business doesn’t require real-time operations today, digital is driving the technology infrastructure to become so. Data interactions with mobile devices, the expanding role of the Internet of Things (IoT), and operations automation are here to stay, and businesses need to operate in real time to support them. Increasingly, the digital world is one of events and interactions that originate outside of a business and require in real-time responses.
The role and activities of business operations will have to adapt to the demands of digital business, adapt quickly to changes in customer behaviors, competitors, and regulations. “Sooner or later, every company trying to go digital will run headlong into roadblocks, likely due to legacy IT. Companies have discovered that their legacy IT is not ready for digital.”8 IT work processes and practices are not fast enough for digital business.
Next-generation applications and analytics need to be designed for real time operations, using consistent and correct data and rules throughout the business, and simplifying the process to change or enhance them. Designing for real-time operation is table stakes for digital business. The fundamental approach to real-time operations is this: every interaction with a company’s applications, mobile, web, or other, needs to use the same data content and rules for real-time and consistent business operations.
IT work processes and practices are labor intensive and time consuming. They need automation to overcome the obstacle of legacy IT with user-, data- and rules-driven capabilities.
Real-Time Data Operations and Automation Action Items
Purpose
Develop next-generation digital business applications that operate in real time, use consistent and correct data and rules throughout the business, and are easy to change or enhance
Action Items
- Establish a ‘data significance’ approach to application considerations so the effects of data inconsistencies, processing latency, and integration, and the additional IT work associated with them, on real-time data interactions are part of the decision process
- Make governance of data, rules, and data use part of the ‘data significance’ approach, occurring before application or IT work takes place and enforcing data consistency and correctness
- Automate application development with a user interaction-, data-, and rules-driven technology to implement next-generation applications that use data and apply rules uniformly
Good data, like good fuel, accelerates a digital business and keeps it running at top speed. Its fuel lines are applications, data streams, and event-based interactions. Each of these needs to be designed for delivering data in real time, and IT operations need to become real-time as well.
RCG can help you achieve data-driven success and increase the efficiency, efficacy, and agility of your business using the latest technology for scalable and sustainable solutions and results that make a difference in your bottom line. To discover how you can achieve your digital and data-driven goals, contact me at Richard.Skriletz@rcggs.com.
Works Cited
1. Why Is It So Hard to Become a Data-Driven Company?, Randy Bean, Harvard Business Review, https://hbr.org/2021/02/why-is-it-so-hard-to-become-a-data-driven-company
2. Bad Data Handbook, O’Reilly Media, Inc., https://www.oreilly.com/library/view/bad-data-handbook/9781449324957/ch01.html
3. What’s Your Data Strategy? Leandro DalleMule and Thomas H. Davenport, Harvard Business Review, https://hbr.org/2017/05/whats-your-data-strategy
4. Data Management, Gartner Information Technology Glossary, https://www.gartner.com/en/information-technology/glossary/dmi-data-management-and-integration
5. What is data architecture? A framework for managing data, Thor Olavsrud, CIO, https://www.cio.com/article/3588155/what-is-data-architecture-a-framework-for-managing-data.html
6. Microservices, Wikipedia, https://en.wikipedia.org/wiki/Microservices
7. Pattern: Microservice Architecture, Microservices Architecture, https://microservices.io/patterns/microservices.html
8. Overcoming Digital’s Biggest Obstacle: Legacy IT, Rudy Puryear, Steve Berez and Vishy Padmanabhan, Forbes, https://www.forbes.com/sites/baininsights/2017/04/26/overcoming-digitals-biggest-obstacle-legacy-it/?sh=69825de66721
https://xkcd.com/
Download a PDF version of this guide by filling out this form
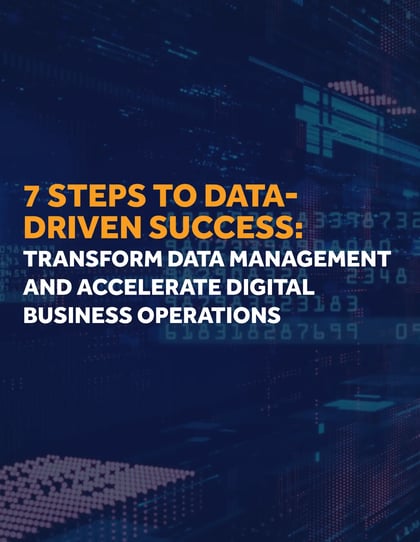
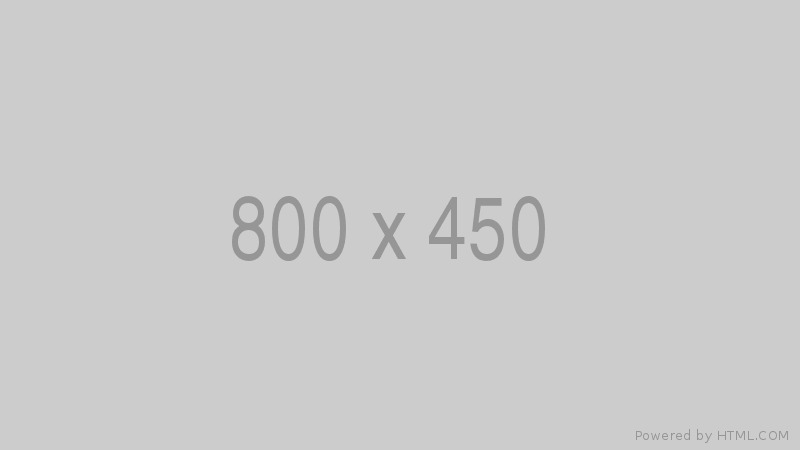
Lorem ipsum dolor sit amet, consectetur adipisicing elit. Ea odio itaque eveniet obcaecati placeat corporis, debitis ipsa nihil cupiditate maxime culpa eaque perferendis veniam.
Joe Smith – Title or company name