Hyper Personalization: Connect To Your Market Of One
To become more efficient with market spending, hyper personalization techniques can tailor a uniquely individual message by leveraging data mining, machine learning, and Artificial Intelligence-based models.
Connect To Your Market Of One
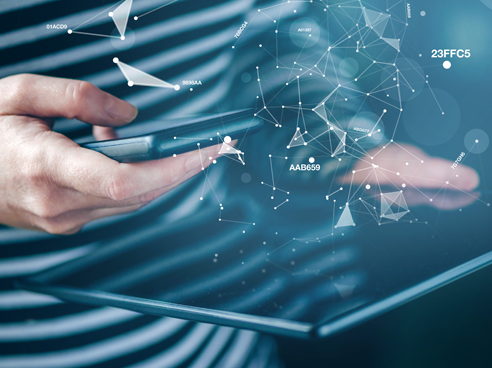
With the bombarding of messages, consumers quickly become overwhelmed and tune out the noise. To capture the consumer’s attention, marketing personnel move to flashier, louder and zanier marketing resulting in a significant investment of resources for a relatively low return rate. For example, I just received an offer for a coupon for a 25% rebate on my car’s tires. My current car’s tires are coming to the end of their service life, and it would make sense to take advantage of the discount and replace the tires early. I called the dealership for additional details and found out the rebate does not apply to my specific tires. The message achieved the goal of catching this consumer’s attention and having them act but at the same time also annoyed them. To become more efficient with market spending, hyper personalization techniques can tailor a uniquely individual message by leveraging data mining, machine learning, and Artificial Intelligence-based models. To extend the example, if the advertiser had been aware of the details of my car’s tires, service history, and purchasing habits, they could have tailored a very impactful message and gained a sale. They could have sent a message that says, “Your car tires will need replacement soon to ensure safe operation in the snow and ice, and if you pre-book your appointment now, we can provide a 25% savings.” If the advertisement included a link to schedule my appointment with at home pick up, I would have purchased the tires early even if I thought the existing tires were okay.
What is Hyper Personalization?
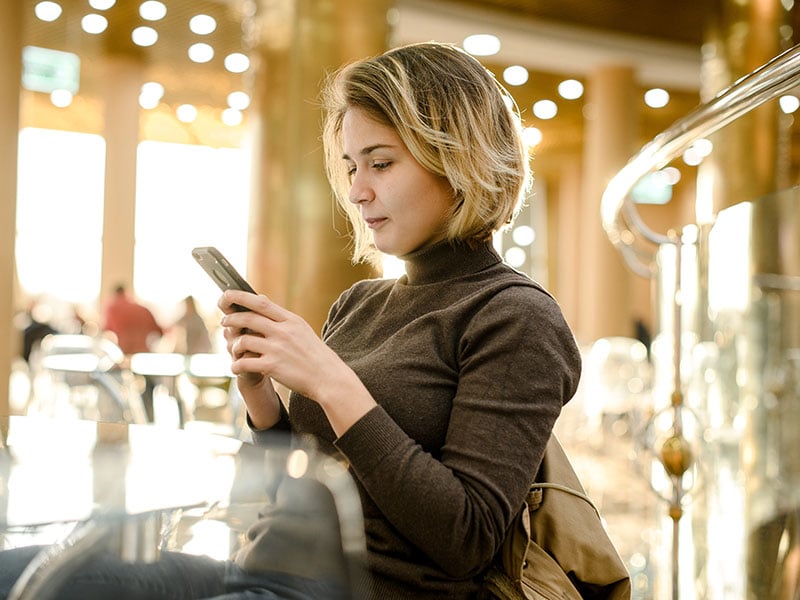
Before we talk about hyper-personalization, let us examine the generally available personalized digital experience. When the customer logs onto a website, they are greeted with their name, prior purchases, and a group of generalized recommendations based on factors such as age and location. The system will use a typical design of a web page with a rotation of available content.
Hyper personalization takes the digital experience from talking to the customer as a group of, let’s say 40-year olds that live in the city with a prior shopping experience to John Smith as the person. No longer are customers put into large groups, they become a group of 1. The digital experience dynamically builds a unique journey blending many factors, including browser history, device type, application of historical data based on the individual customer persona, location, purchasing history, propensity to purchase other products, etc.
What is hyper-personalization? Hyper-personalization is an advanced and real-time customization of offerings, content and customer experience at an individual level. Designed to perfectly match a customer, hyper-personalization leverages Big Data to deliver such tailor-made solutions in real time.
Customer expectations Consumers have experienced hyper-personalization through digital-first companies like Amazon and Netflix and are now extending those same expectations to their other digital experiences. Marketo reports that more than 85% of the consumers expect and accept personalization as a part of their online retail experience.
According to Forrester, over 77% of the consumers have chosen, recommended, or paid more for a brand that offers a personalized service or experience. According to a 2019 McKinsey study, organizations that have implemented personalized recommendations and triggered communications have realized a 5 to 15 percent increase in revenue and a 10 to 30 percent increase in marketing spend efficiency.
When companies can provide a rich hyper personalization experience, revenues increase.
Elements for Hyper Personalization
When these elements are combined correctly, marketing messages can be delivered to the customer at the right moment in the purchase journey and tailored to the specific customer’s motivations and desires. For example, a customer is walking in the general vicinity of a restaurant around lunchtime. Hyper personalization will provide a message like “We enjoyed serving you our juicy burgers last week, stop in today for another great burger and enjoy dessert on us” could be pushed to the customer’s phone within eyesight of the restaurant.
Using the details of geography, demographics, and shopping behavior, a personalized message can be delivered, which, according to Epsilon, over 90% of customers find appealing.
Progression to Hyper Personalization

- The vast majority of today’s companies provide the basic personalization experience as outlined in the green box. Their messaging includes providing a single message such as a flyer, email, or mailer to a segmented customer group. For example, a retailer could send a brochure out with a discount code to any person who purchased a product in the past six months in the specific zip codes. Companies progress through six significant phases as they become a skilled expert in providing a world-class hyper personalization experience.
- The next step would be to make the flyer personalized with a mail merge, such as “We noticed you purchased insert type of item last month from your insert store location. Please use this coupon for your next purchase.”
- The next level of personalization involves multiple types of messaging based on general customer demographics and history. Throughout this process, the company uses broad strokes to drive customer segmentation and provide a simplistic personalization based on the large grouping. The end result of this type of personalization is akin to receiving a form letter where there is an attempt to make the customer feel unique, but it is evident that the customer is just a number.
- The next step is behavioral recommendations, making offers based on how the consumer interacts with the digital experience. Some examples include the consumer’s browsing, purchase, and cart non-purchased history. Based on these values combined with static data elements of the consumer, can send a semi-customized such as, “Insert Name, yesterday you were shopping for a new drill. Today is a great day to finish your purchase!”
- Behavioral recommendations are very singular channel-focused, such as the consumer’s digital experience or talking with an agent. Omnichannel optimization looks to build a complete customer profile based on all interactions on all channels. This would allow a consumer to start a transaction in the digital experience, chat with a representative to answer a question, and walk into a physical location to complete the transaction. The transaction would be seamless to the consumer after providing an identifying piece of information such as a log on, id, etc. However, to the company, it is managing the customer journey across multiple access points. The focus of hyper-personalization is not to develop or implement an omnichannel solution but rather provide personalization along the journey. For example, when the consumer is chatting, the company can attempt to cross-sell a complementary product to the transaction. When the consumer is in the physical location, the company can understand what prior offers were accepted and rejected so the same ones are not repeated, or a new one is provided. The end goal is to understand your consumer, where they are in the journey, provide targeted messages to either cross-sell or ensure the sale closes and the customer receives a personalized experience.
- The final step in the hyper-personalization journey is to implement predictive personalization. This capability addresses the company’s ability to predict what the customer will need before the customer realizes they need it. This is based on a large volume of data from comparable consumer experiences driven through a complex model updated in near real-time.
Making Hyper Personalization a reality
The idea of hyper-personalization is a fantastic concept. Offering the customer a unique message seems simple, but to build the technology, methodology, data store, and infrastructure is not a small feat. To be successful, the approach must be one that can support the daily terabyte deluge and efficiently dissect the deluge for trends and present back meaningful offers to the customer. All of this work must be done in near real-time as the consumer habits and experiences are ever-evolving based on events of the day
The company must build a depth of knowledge of its own products.
To create a uniquely tailored offering to the individual, companies must build an intimate knowledge of the products being offered. Today, most companies understand the segment of the product, say kitchen cleaning products. They may even drill down to the next level and break it into the next level of the type of cleaners such as stainless, tile, wood, and glass cleaners. Using this level of detail is not sufficient for the hyper-personalization as the recommendation would result in four or five different glass cleaning products. Instead, knowledge must be gained and tracked on an individual product level.
- Product details
- Surfaces the product cleans
- Ingredients/type of cleaner such as vegan, non-chemical, etc.
- Use of the cleaner such as commercial versus personal
- Purchase Trends
- Type of people that viewed the product
- Types of consumers that last purchased the product
- When it was purchased last
- What was in the shopping cart when it was purchased last
- When was it removed from the shopping cart
- What other types of products has the consumer bought in the past
- Reviews
- Rating of the product
- Rating of the vendor
The product details can be easily captured from the various manufacturers and uploaded as a grid for the consumer to filter and sort after the recommendation has been made. The more difficult challenge is developing the purchase trends. Most companies will track who bought the product upon check out and maybe if it was left in the cart, but the finer grain of detail of the consumer, time of purchase, etc., are usually not captured. The concept of gathering the fine detail of when the product was viewed and purchased may sound a bit farfetched, but this is a critical component of increasing the sale probability. For example, marketing companies have been able to determine the best time of day to send emails based on when they open the email. The same applies when selling the product. You may find the commercial shoppers are filling the purchase request from the overnight cleaning crew in the morning. You will quickly realize they order what is on the list, but if you suggest glass cleaner in the morning, it will sell better than in the afternoon when it is better to push candy.
In addition to gathering the purchase’s timing, the company must capture additional details of the purchaser. This would include unique attributes such as the purchase location, the device being used, cookie history, email, and other available metadata. This data will build a historical profile of the purchaser during the digital experience and use as data to predictive models for other similar purchasers.
Data must be gathered about the customer
Companies generate mountains of data daily, but most likely, it is in siloed systems that do not communicate with the enterprise. The data will be at different granularity levels and most likely have differing meanings for the same concept, such as what is a customer or what is a transaction. For hyper personalization to work, the point-of-sale system, digital experience, customer relationship management, and inventory systems not only need to speak and understand the same language but must transact in real-time. For example, a customer is suggested an offer for a product based on their profile, but the product is not available when the customer goes to check out. Not only has a possible sale opportunity disappeared, but it also created an angry customer thinking, why are they asking me to buy something they don’t have?
Data capture does not only happen in internal systems or third-party updates based on a transaction. However, it can be easily captured from the customer when they are digitally interacting with the company.
When customers come to your sites, they bring the history of their Internet browsing through cookies, which can be read and stored in the company’s systems. Other technologies will enable the capture of IP addresses, types of devices, and other passive details. Using this data, third party data services can enrich the data to provide a more complete picture of the customer. In addition to passively collecting information, a company could simply ask for it. According to Inc.com, 61% of American consumers are willing to share data with a company to receive a more meaningful and personalized experience. This could be done through surveys, registering for special promotions, receiving emails, or the checkout process after purchasing a product. Access to consumer data must be taken with an increased focus on security due to the ever-increasing strictness of privacy laws and customer’s distrust of how the data is used.
Storing and Accessing the data can be more important than capturing it.
Capturing data is a relatively simple task, but storing and accessing it can prove to be challenging. When storing the data in the data warehouse or data lake, careful consideration must be given to how the data will be used. The data will need to be stored, accessed, and updated in sub-second response time to enable real time offer presentations by AI. The machine-based learning model used by the AI will also require near-real-time interaction to make continual updates. Also, marketing, data, and other personnel will need to access the data to perform analytics. As a result, careful thought must be put into the naming of columns, the table structure, and even how the data is stored as it will be gathered from multiple sources.
Build an AI model to harness the data river
Before harnessing the rivers of data, a detailed predictive machine learning model will need to be built. The model will consider a variety of apparent elements such as age, location, etc. but also account for other elements such as time of day, currently trending topics, user cookies, type of device, etc. The model cannot be one that is static but instead reinforced with machine learning techniques to allow the model to grow and shift over time. The flexible models are the ones that propelled retailers into growth during the Covid-19 Pandemic as they quickly adapted to new customer behaviors without a complete rewrite or human intervention for a significant correction.
Once the data rivers are flowing and the model has been established, AI will play a critical role by understanding the customer’s metadata in real-time, understanding the customer current purchase intention, reviewing the results of the model for most likely to buy offers, understanding the availability of the product and selecting the best option of many to present to the customer. In addition, the AI must also account for what is currently in the customer’s cart, what the customer has browsed but not purchased, and the duration of browsing during the digital experience. These actions must be done in the time it takes to present the next page to the customer.
Companies to Model

Your competition is no longer other companies in your segment, but rather any company across any segment. When consumers have an impressive digital experience, their expectations have now been raised when they interact with other companies. Consumer’s experiences include one-click order, ease of searching, chat features, and other capabilities that they see as simple and easy. The consumers then apply those expectations to other company’s digital experiences. As technologists, we understand it is not as simple to add a chat feature to the web page due to security concerns, data storage, implementation, architecture upgrades, etc. The consumer is only interested in the experience, and if they are not satisfied, they will go to your competition.
Amazon
Arguably, Amazon is the most proficient at hyper personalization in the digital arena. They started the efforts in 1998 with Amazon. com collecting data. Since then, Amazon continues to add data and expertise through additional data collection portals such as Kindle, Alex, Wholefoods, etc., to build an ever-increasing data set and experienced model.
Amazon uses a recommendation engine algorithm that is called, item to item collaborative filtering. The algorithm uses elements such as customer demographics, search query, average time spent on searches, past purchase history, brand affinity, category browsing habits, time since last purchase, the average purchase amount, products purchased by other similar customers, and other proprietary factors.
For example, when a consumer searched for an olive-green pair of running shoes and did not purchase the product, Amazon creates a hyper-personalized email recommendation of alternatives that may be a better fit. In this example, the AI determined it was better to offer shoes in different colors and price points.
Amazon not only uses this information for making suggestions on a purchase but for the supply chain logistics. Big Data analysis shows that customers will shop elsewhere if the product is not in stock and promptly delivered. Some customers are even willing to pay a premium for a product that is in stock, as shown through the recent Covid-19 pandemic. Amazon uses the recommendation suggestion analytics to preplace products in local distribution centers close to the probable consumer. This enables higher sales while lowering delivery costs and reduction of delivery time.
Amazon has harnessed hyper personalization efforts to provide a thirty-five percent conversion rate on the message sent. Amazon has also been able to productize the hyper personalization efforts for other companies to plug and play through its Amazon Web Services platform.
Starbucks
Starbucks has developed an internal program called Digital Fly Wheel that outlines its digital experience strategy. Starbucks uses an AI algorithm to send over 400,000 variants of hyper-personalized messages through push and in-application notifications.
Starbucks uses a unique combination of contextual data, such as application location, customer demographic, customer activity, and past purchases, to develop an individual offer. The Digital Flywheel’s impact has been a threefold redemption of offers, with an estimated twenty-five percent of transactions being conducted via the application.
Netflix
Netflix has a plethora of movies, series, TV shows, and other entertainment titles available upon demand. Netflix’s challenge is keeping the over 180 million global subscribers engaged in continuing the subscription and not get lost in the sea of options. The average subscriber is willing to invest up to 90 seconds, searching for an option before the user loses interest. To keep the subscriber engaged, Netflix has developed an algorithm to predict the subscriber’s interests based on three key elements, customer behaviors, entertainment metadata, and other subscribers’ viewing habits.
Netflix can combine this data and present a set of recommendations to keep the subscriber engaged and deliver it uniquely to each subscriber through a highly contextualized and hyper-personalized home page. This has resulted in eighty percent of the subscribers selecting the recommendation while twenty percent perform searches.
The Power of Hyper Personalization
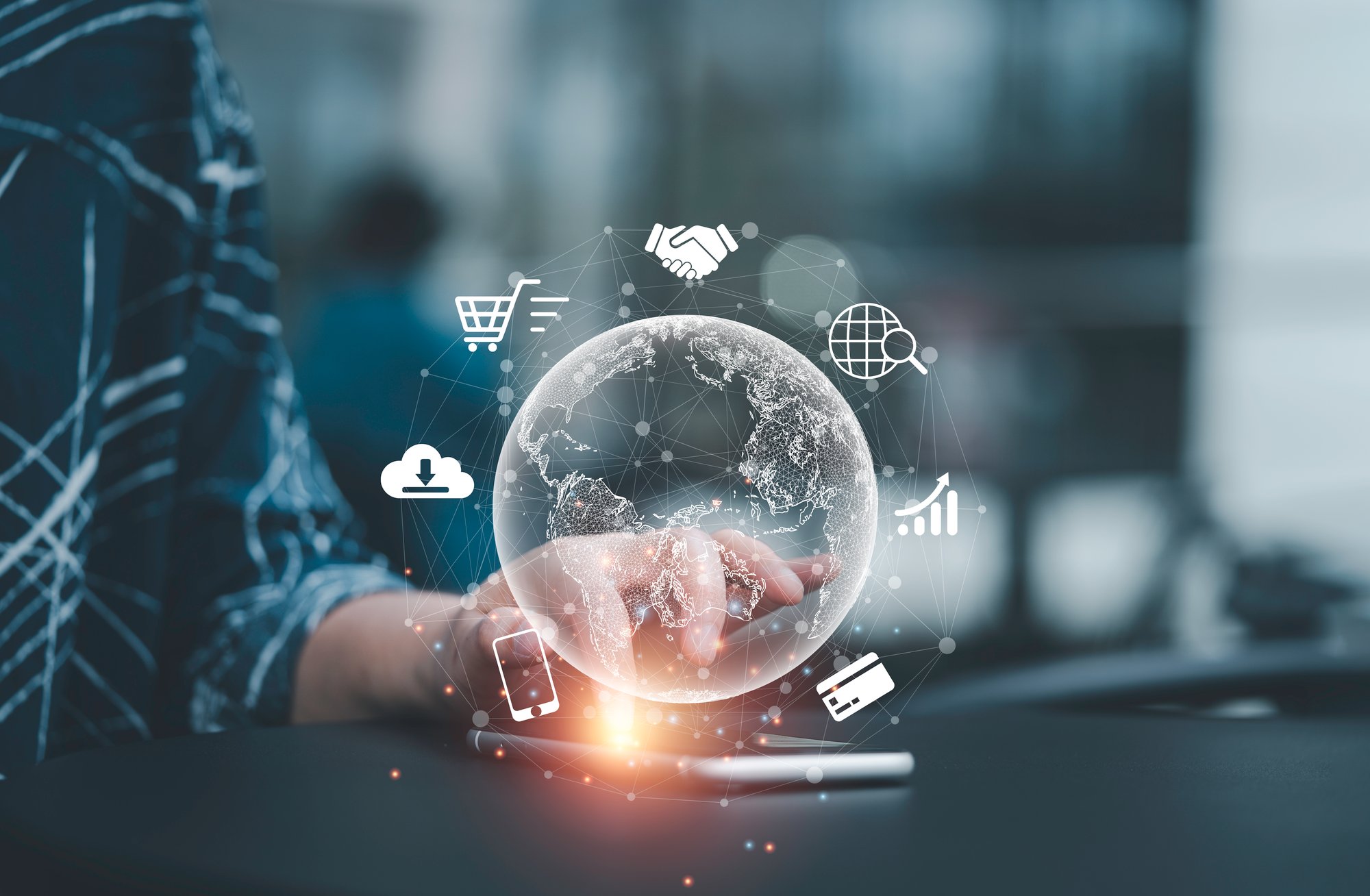
Building the capability of hyper personalization is no small feat. It requires significant efforts to know your product catalog, builds messaging, capture data about your consumer, and build detailed models to predict the consumer’s behaviors. Achieving the goal requires more than analytics. It also requires infrastructure and architecture changes from providing sub-second response time to access, review and analyze data, ensure all systems are gathering the data and placing it into a centralized data store, updating existing digital experiences to increase data capture and accept inbound messaging. Each effort in the journey appears to be simple and easy to accomplish, and individually, the work is simplistic. Once the program view is applied, and data is examined holistically, the complexity increases dramatically as data needs to serve multiple purposes and systems built in different eras need to consume and interact with data in new ways. Making a move to hyper personalization is the way to support future sales growth but will require a distinct focus on delivering.
The journey may be difficult, but with RCG as your partner, we will create a world class digital experience.
Download a PDF version of this ebook by filling out this form
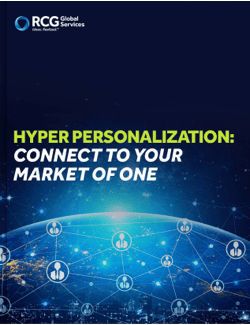
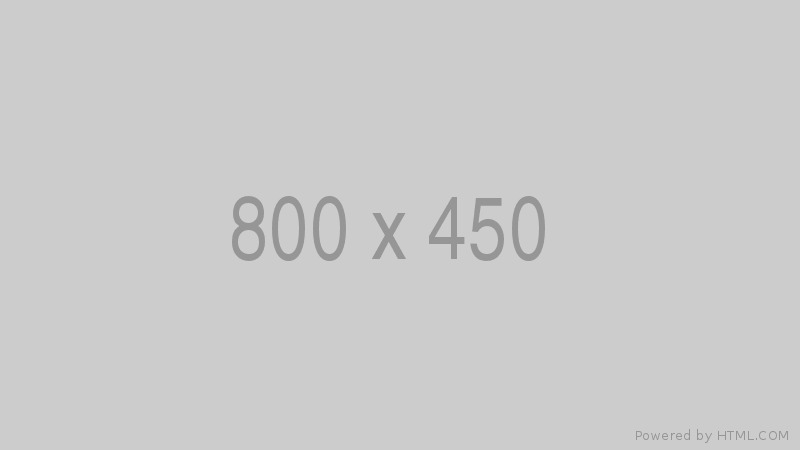