Four Fundamental Obstacles to Successful Analytics
Executive Summary
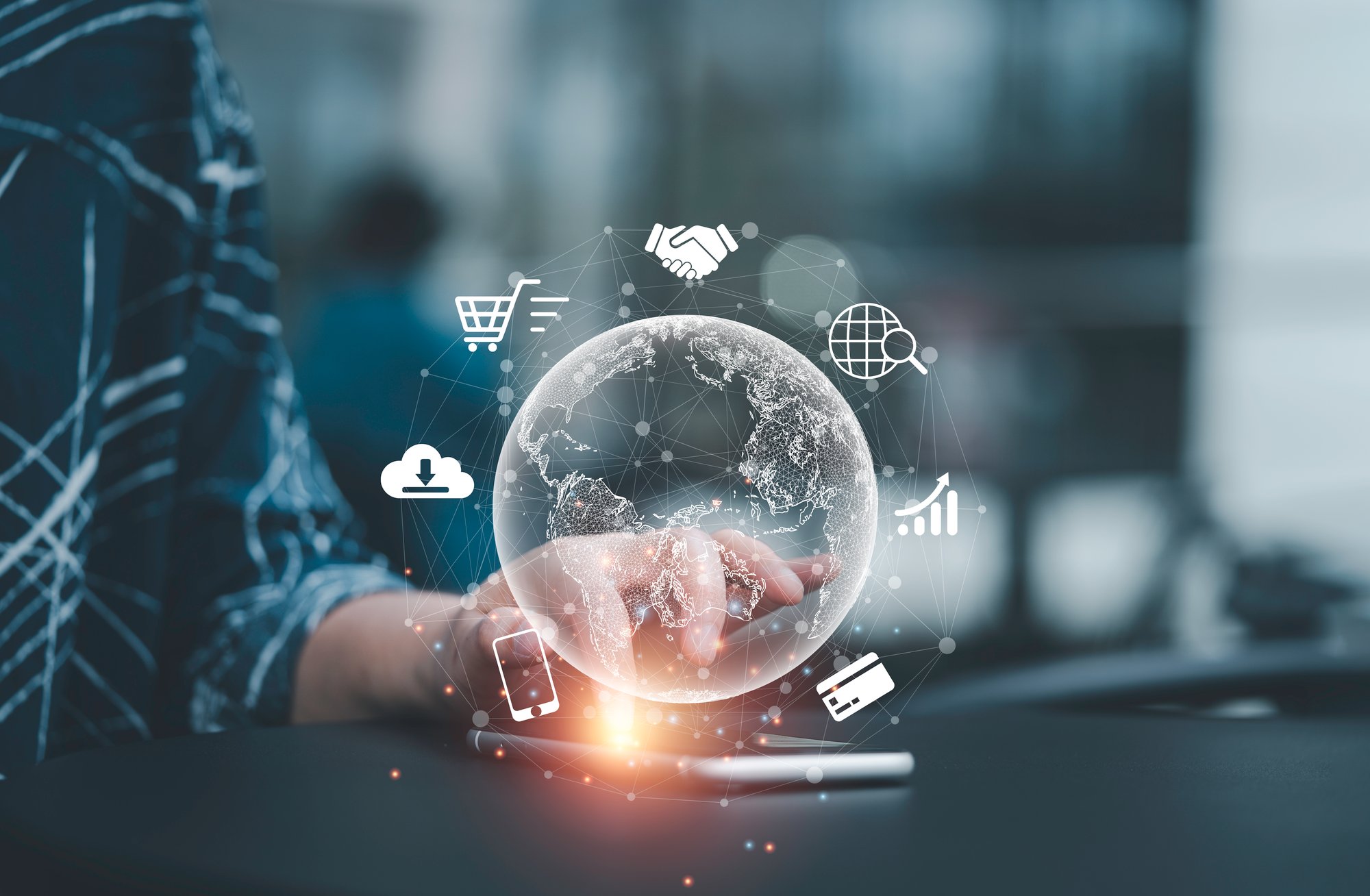
For many years, an ever-expanding number of business intelligence tools have promised to fully satisfy the needs of decision makers. But that promise has gone unfulfilled due to key obstacles that must be addressed for success with the newest technology for analytics: Big Data.
Four fundamental problems have prevented companies from fully realizing the promise of pervasive analytics.
The first is a fundamental failure to deliver data to business users that is ready to use without further manipulation on their part for decision-making and analytics.
The second problem is the difficulty of using existing business intelligence tools, especially for non-technical business users. Usability is a huge obstacle for user self-service, especially tool consistency across the wide range of data uses, from reports and dashboards to ad hoc queries and predictive analytics, required by the business.
The third is poor execution in the preparation, management, and delivery of data. Business intelligence technologies have evolved over two decades, yet few, if any, businesses have developed a reliable foundation of trustworthy data to meet the data demands of the business.
And the final problem is the high cost of conventional business intelligence and analytic tools, particularly when scaling them to enterprise-wide use.
Emerging technologies, like earlier business intelligence technologies, promise to finally fulfill the long-standing promises of the Information Age for available, ready-to-use, and real-time data and analytics. Open-source technologies such as Hadoop® can capture all types of data including live, structured, semi-structured, and unstructured, and process them in real-time. These technologies enable a faster, more efficient capture of data, lower-cost data storage, and increased analytic capabilities.
But achieving available, ready-to-use, and realtime data and analytics with these technologies requires overcoming the four obstacles identified above. This begins with an 'outside-in' rather than an 'inside-out' view of data management.
‘Outside-in’ means understanding all business data use scenarios first so the design of a data solution and selection of data technologies can be confirmed to support all of the business’s use of data. This is a holistic rather than an ‘inside-out’ view that designs a data solution and selects data technologies with a limited number of data use scenarios, often for a single department.
Promises Unfulfilled
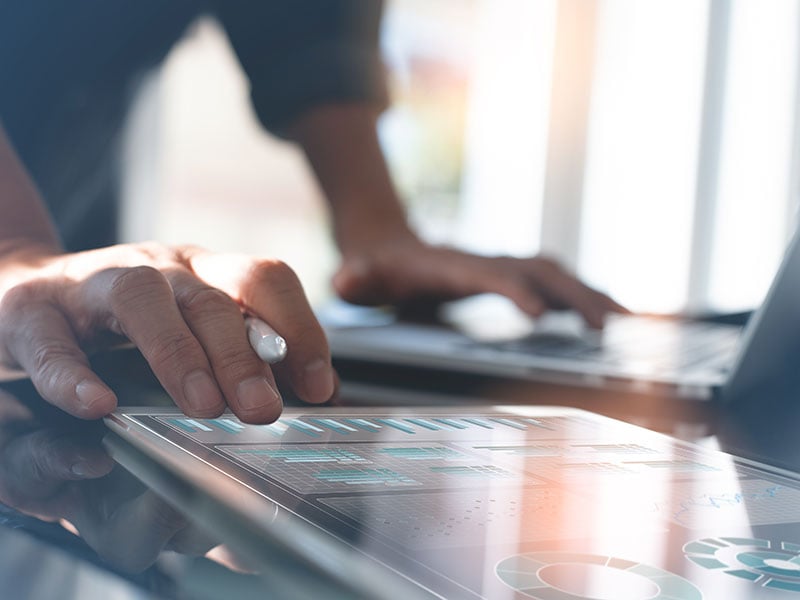
All along BI has seemed a very promising technology, offering the potential of fulfilling the two most essential needs of business users: providing data as needed, when needed; and providing a unified, organization-wide single-version-of-truth.
Unfortunately, business intelligence has not fulfilled either of those needs.
That failure hasn't been for lack of effort. For more than 20 years, software providers have been creating and fine-tuning new BI tools, each promoted as finally delivering upon the promise of BI. And like hopeful seekers drawn toward a vison of the Holy Grail, business users have continued to spend big bucks on BI in a never-ending quest to finally realize the promised potential.
The unfulfilled promise of BI has left a gaping need that business users have attempted to fill by resorting to "shadow IT": "Just give us the data; we'll do the best we can to adapt it to our needs." And this approach has carried organizations even further from the single version-of-truth Holy Grail by encouraging the creation and proliferation of data silos.
Is User Self-Service Really a Problem
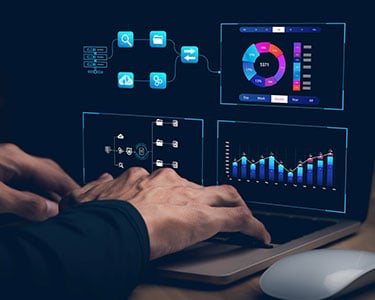
Why is this a problem? Because executive management is spending time trying to make the data usable for their needs.
That leaves them with less time for analyzing situations and making sound business decisions - their very reason for needing BI. Decisionmakers are left with less time to perform their jobs, because they're spending time, in effect, doing IT's job. BI's failure to deliver upon its longstanding promise is rooted in the four following problems.
Problem #1: You Can't Trust Your Data
Many analytics and business intelligence tools have been installed at thousands of companies around the globe. These tools are relied upon for the delivery of actionable analytics that will support quick and accurate decision-making.
But there's a fundamental flaw that prevents these tools from delivering upon their promise: the quality of data that's provided to the tools. It's a problem that hearkens back to one of the oldest clichés of the Computer Age: garbage in = garbage out.
Most business executives believe the data in the systems they use is sound. Indeed, the data found in storage at most businesses is perfectly suited for traditional uses such as generating invoices, processing payroll, and performing a plethora of routine business chores. But that same data doesn’t provide reliable analytics and insights for all data use scenarios the business needs without significant data preparation.
And yet it's quite commonplace throughout the business world for 'cutting-edge' analytics tools to be installed and put to work with little thought about all of the ways the data will be used. After all, businesses have been using computers and databases for decades - and much of the data in storage has been in place for just as long. And that leads to an attitude of: "Our data is our data. It's what we have available to use. It's what we've always used."
Looking at Data: Inside-Out vs. Outside-In
Traditional application data is designed to support business transaction processing, not the wide range of data-use scenarios demanded by today's business needs. And that reveals a fundamental flaw with many business intelligence solutions. To a large degree, data for business intelligence reporting and analytics resides in a data warehouse or operational data store. It's the traditional data that has long supported standard software applications, and making it available for other uses is important.
However, two flawed data development practices have been common.
- The first is to extract data from source applications and structure it in a particular way based on the “philosophy” or school of data architecture preferred by the architects.
- • The second is to extract source data and shape it to meet the needs of a particular business need, such as a dashboard, without considering other data use scenarios. These approaches are 'inside-out', making application data usable for a limited number of data use scenarios.
There is a better approach. Data requirements should be analyzed from the 'outside-in.' Begin by understanding all uses of the data needed by the business today. The purpose of each data-use scenario must be analyzed to determine the suitability of existing data sources, the underlying data structure(s) needed, the data technologies required, and the operational processes necessary to develop and sustain a foundation of trustworthy data.
Taking this approach is likely to reveal gaps in internal data that require supplementation from outside, third-party sources, technology, and operational and organizational capabilities required for success, especially as user analyses begin to include text, image, video, sensor, and other Big Data data types.
Developing a foundation of trustworthy data requires Business Data Management, the business-led process for data governance and master data management. After all, for data to be trustworthy requires the business to establish the rules about data including its definition, its source, its transformations to standardize and conform to the rules, and so forth. The outside-in approach requires that Business Data Management establish the definitions of data elements and the rules that apply to them so that business users can understand the data they are using and answer questions for themselves about what the data is, where it came from, how it was transformed, and more.
The outside-in approach assures that the data is trustworthy for use in analytics, as well as in all other data-use scenarios.
Problem #2: Your BI Tools Are Hard to Use
BI tools are intended to deliver actionable insights to non-technical people, most often in management or executive positions. And yet many BI tools are quite complex, requiring a sophisticated level of technological prowess from users.
The result, of course, is that the intended users of business intelligence tools shy away from the steep learning curve associated with getting useable results from these tools when they are available (see Problem #4). Instead, many users revert to using more basic tools such as Excel or Access.
But BI users can essentially be divided into two camps: data consumers and data analysts. Data consumers simply need the ability to navigate to a report, analysis, or dashboard display that has already been prepared for them. Data consumers require an easy-to-use interface to access the data objects they need to see. While many BI tools today offer web interfaces, they can be challenging to navigate and integrate into company portals.
Data analysts need the ability to create their own report, analysis, or dashboard. Here again, the interface needs to be straightforward, however data analysts generally like to master the tools of their trade. Many BI tools require an understanding of physical data structures in the data warehouse or operational data store to access and use data. What is needed is to manage data access through the data definitions established by Business Data Management processes.
Although BI tools are often attractive to endusers because of their ability to deliver goodlooking reports and dashboard displays, most do not adequately support all data use scenarios the business needs, requiring multiple BI and data tools for users to understand and use.
Problem #3: Your Organization Can't Properly Execute the Preparation, Management, and Delivery of Data
A separate issue from the plethora of business intelligence tools is the handling of data. Most organizations have failed to develop the disciplines, infrastructure, and processes required for developing and sustaining a foundation of trustworthy data designed to support all data use scenarios the business needs.
Many businesses say they treat their data as an asset, but few follow up with the operational and Business Data Management processes to manage data. Everyone recognizes the need for a ‘single source of truth’ as a foundation for satisfying business information needs, but few make it happen. There are many reasons for this, primarily the way IT projects are funded and managed combined with a lack of accountability for the data content and support for business data use scenarios of an enterprise data warehouse or operational data store over time.
This problem will be discussed in detail in a future white paper.
Problem #4: You Can't Afford Your BI Tools
In many instances companies simply can't afford to implement business intelligence tools throughout the organization. When BI tools were first introduced a couple of decades ago, they were premium-priced tools. And the passage of time has done little to lower the costs of implementing these tools.
The costs of scaling BI tools to an enterprisewide level - including training, licensing, and support - are often prohibitive. Tension often arises between vendors seeking to maximize revenue realized from their data and analytics products, and companies straining to afford the costs of implementing the products across the entire organization.
Open source technologies provide an opportunity for implementing BI and analytic tools at far more manageable costs. While open source BI tools began appearing over 10 years ago, the arrival of open source data platforms like Hadoop has accelerated the development of open source analytic tools that hold promise for scaling to an enterprise level at a lower cost
Achieving enterprise success with data requires solving these four problems:
- delivering data and analytics on demand;
- usable tools for all users;
- effective trustworthy data management practices, processes, and disciplines; and
- affordable costs for today’s world of data and analytics.
Analytics for Today's World
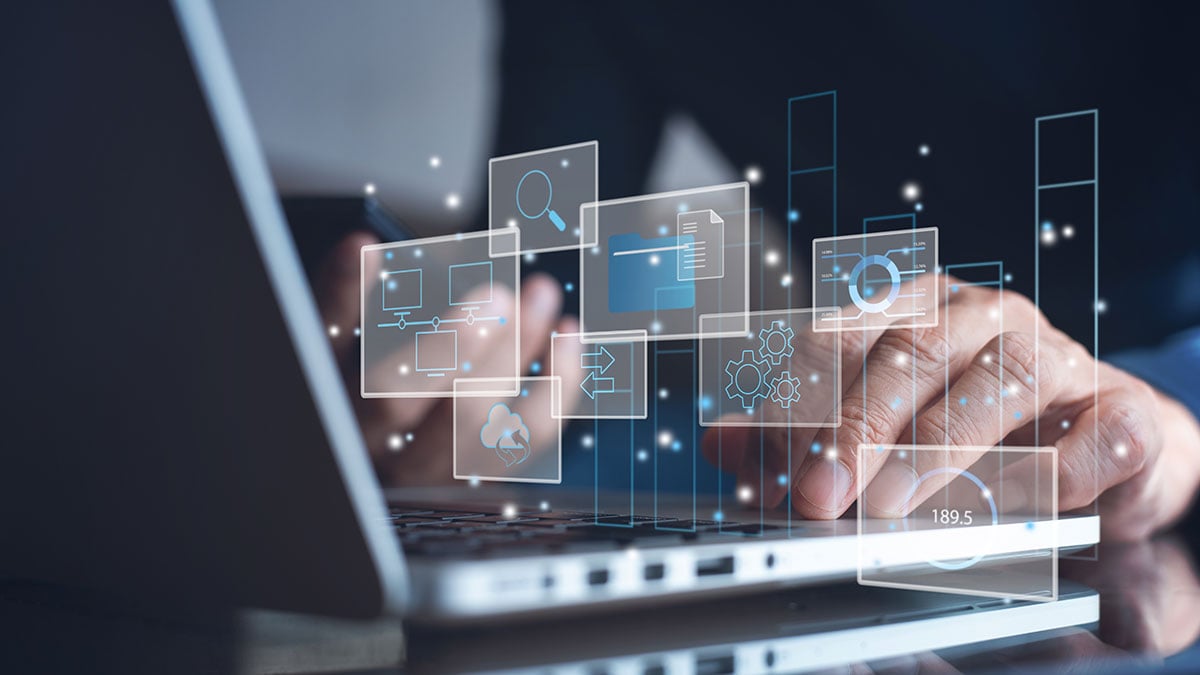
Analytics must evolve accordingly. The existing paradigm must change to support users interacting with data in highly mobile, on-demand environments. Consequently, there's a move underway toward open-source solutions that are scalable, mobile, and interactive.
For any organization, impetus toward data solutions that deliver analytics and insight can begin with one important step: developing an Information and Analytics Strategy.
Developing this strategy will identify all data-use scenarios needed in the business. It will spotlight areas of opportunity for the implementation of analytics and their potential value. It will identify the technologies, skills, disciplines, processes, and organization needed for success. It will develop a roadmap presenting a sequence of projects each of which delivers business value.
In short, developing this strategy will help to reveal the technologies and disciplines needed to finally realize the long-promised benefits of analytics and plan the foundation for text, image, video, sensor, and other Big Data data types.
For companies that have been dreaming of the Holy Grail of on-demand data delivery and analytics, an Information and Analytics Strategy offers an opportunity to finally set the stage for fulfillment of the dream that Big Data technologies promise.
Notes
1. Columbus, Louis. "Key Take-Aways From Gartner's 2015 Magic Quadrant For Business Intelligence And Analytics Platforms." Forbes, 25 Feb. 2015: n. pag. Web. 16 Aug. 2015.
2. Bertolucci, Jeff. "Big Data ROI Still Tough To Measure." Information Week, 29 March 2013: n. pag. Web. 16 Aug. 2015.
3. "Gartner Says Consumerization of BI Drives Greater Adoption." Gartner. Gartner, Inc., 20 July 2011. Web. 16 Aug. 2015.
Download a PDF version of this whitepaper by filling out this form
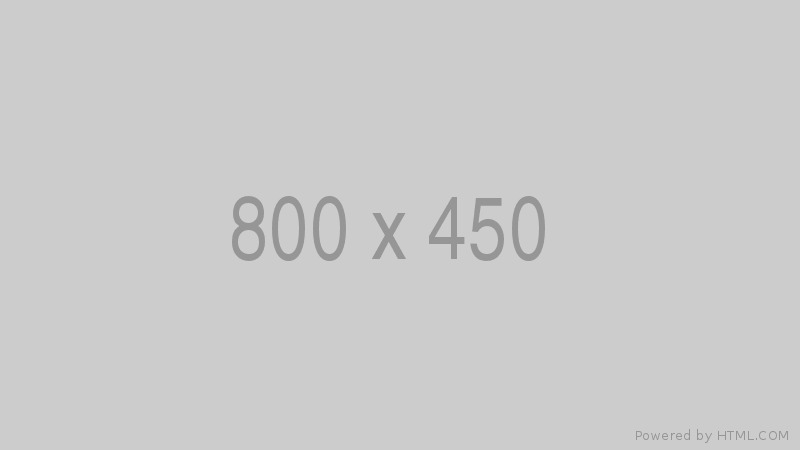